94% of fake AI exam submissions went undetected — University of Reading
Wednesday 4th December
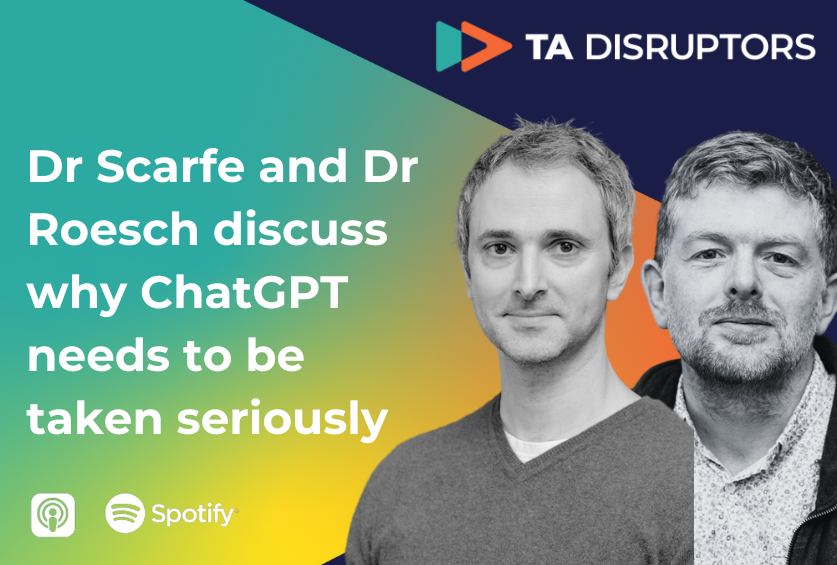
Since the COVID pandemic accelerated reliance on unsupervised ‘take-home exams’, the education system has found itself with a fundamental problem –– one which has only been exacerbated by the recent rise in easily accessible AI tools like ChatGPT. And new research shows just how great the scale and impact of the problem is for the education system and Early Careers teams alike.
Dr Peter Scarfe, Associate Professor, and Dr Etienne Roesch, Professor of Applied Statistics & Cognitive Science, both at the University of Reading, are two of the minds behind a recent groundbreaking study which proved just how easy it is for students to not only use AI in exams but to outperform real students. And most importantly –– evade AI detection in the process.
In this week’s episode of the TA Disruptors podcast, Martyn joins Robert as they discuss:
Join Robert, Peter and Etienne for the first episode of our new season of the TA Disruptors podcast on the impact of the AI-enabled candidate on recruitment. Our guests dive into:
💡 The details behind the study in which 94% of fake, ChatGPT-generated submissions for five modules in a BSc Psychology degree went completely undetected (and performed half a grade higher than the average student) –– even with simple copy-paste answers and no advanced prompting
🤖 Why detecting AI usage is notoriously difficult and what this means for universities, Early Careers teams, and hiring managers when it comes to ensuring their assessment process is fair, accurate, and maintains its integrity
🎓 Why reverting to traditional invigilated exams or proctored assessments isn’t a sustainable solution –– and how universities are already ahead of employers in rethinking their assessment process to embrace AI rather than treating it as a threat.
🧠 What these findings mean for talent acquisition: as graduates increasingly rely on AI to boost their results, how should recruiters and hiring managers adapt their processes to evaluate candidate capabilities fairly?
🔮 The broader implications of AI for education and recruitment, and how we can expect advances in tech to reshape the future of work and learning forever
If you want to understand just how difficult it is to detect AI, both from a human perspective and also a software perspective, and just how severe the implications are for the world of Talent Acquisition and Early Careers recruitment, this is a conversation you won’t want to miss.
Listen below 👇
Podcast Transcript:
Robert: Welcome to the TA Disruptors podcast. I'm Robert Newry, CEO and co-founder of Arctic Shores, the task-based psychometric assessment company that helps organisations uncover potential and see more in people.
We live in a time of great change and TA disruptors who survive and thrive will be the ones who learn to adapt and iterate and to help them on that journey. In this podcast, I am speaking with some of the best thought leaders and pioneers who are leading that change.
I am especially excited to be welcoming today two professors of psychology, Dr. Peter Scarfe, associate professor at the University of Reading, and Dr. Etienne Roche, professor of applied statistics and cognitive science at the University of Reading. And both of you have a PhD in psychology. And I know that you have a particular interest in many different things, but what caught my eye and that of the BBC and the general public was your recently released paper that came out in June where you conducted a fascinating piece of research that I'm sure our listeners will be really excited to learn about, about the use of AI in exams at universities.
And before I go into how you set this up and some of the results around this, I'd just like to set the scene of why this is such an important piece of research and why I think people will find this so fascinating of the things that you uncovered. So since the start of the pandemic, similar in the university exams as to graduate recruitment, people have been relying more and more on take-home style exams rather than invigilated ones and that's as true for university exams as it has been in the graduate recruitment world. And so your paper looks at a real world Turing test and I love the fact that you used that word too because it's something that I think most people are kind of familiar with, that idea of can artificial intelligence trick, outwit, pass as a human in a real-life environment.
And the fact that you've used that in an exam system using ChatGPT to infiltrate the particular course. And that you've done it in a really rigorous way and one that will stack up to review, and I know you've released a paper on it as well. And so the relevance of that is that a lot of people have been talking about AI and it being able to influence exams. Some people have imagined that to be more scaremongering than reality, and it's only when you get a peer review type of research that really digs into this, can we really start answering some of these big questions about what will the impact be of AI.
So on that, let's look at the two big questions that I think your research was looking to address here. Amongst others, but the two big questions I think will be relevant for this discussion is would AI get a student a better result if they used it and we'll talk about how. And then secondly, could a student using AI go undetected in a real life exam situation? So with that in mind, could you share with us… I don't know which one of you wants to go first and perhaps explain how you set this research up to create some AI students and infiltrate the exam system.
Peter: Well, as you mentioned, there have been a lot of anecdotal reports of educators running their questions through AI, grading the answers and the answers being very good. So we're obviously both scientists and we love our data. And we wanted to just get some hard evidence rather than this kind of rumours and anecdotes. So the way in which we went about that was we entered 100% written chat GPT answers to exam questions into five modules across an undergraduate psychology degree. So from first year through to finalist.
Those questions were submitted by kind of fake student aliases that we got produced. So the key thing with the study was basically to our markers, these just looked like any other student.
Robert: Right, so they had no idea at all that you'd infiltrated the exam process?
Peter: That's key to get hard data basically. If we'd have told our markers of that there might be. The possibility of this study working, our markers might behave very differently and that wouldn't have been reflective of kind of reality. The real, the real situation we're in, yeah.
Robert: And so they didn't, they had no idea about who these were and it covered the entire exam set of modules for a psychology degree or was it just a part of it?
Peter: No, it wasn't all of the modules. We were limited in the modules we could submit to because we needed to kind of compensate for the additional workload basically. So we submitted to five modules in total across all years.
Robert: Okay, so just an exam case, so no coursework involved in it. And in the exam setup, which is, I assume, quite typical, is it of many degree courses at Reading that they had 24 hours, that this is now quite a typical setup of how people are doing exams in any degree at Reading, not just psychology?
Peter: Yeah, so there are two types of exams, essay-based exams, which was effectively kind of like a full day, around kind of eight hours-ish. And then short answer question exams, which were in a shorter window of around two hours.
Robert: Around two hours, okay.
Peter: So on the day, myself, Etienne, and some of our collaborators on the study pretended to be students logged in, and submitted on the exam system.
Robert: Okay.
Etienne: Stressful.
Robert: I bet. So you were having to kind of pretend to be students on this, but literally all you were doing was putting the question into ChatGPT and copy and pasting from that into whatever online application.
Etienne: We had pre-prepared answers
Robert: Sorry, pre-prepared answers?
Etienne: Yeah. So we had used ChatGPT in the weeks prior to the exam because we knew the exam questions already. And so we had pre-prepared a set of questions and so on. And on the day we had to log in with a fake student account, pretend to be the students to download the questions towards the end of the period we had to wait or six hours or I don't remember. Then we had to log in again and submit the responses.
Robert: How much manipulation of the responses did you do?
Etienne: None.
Robert: Because people are just gonna assume, hang on, you're professors, of course you're going to be able to look at ChatGPT, add a slightly better topping and tailing of it, and so naturally, but you didn't do any of that?
Etienne: None whatsoever, I think we would have, but it was such a lot of work that we didn't have time to do that anyway. Yes. And so that's actually a good thing for the study because it demonstrates that even the lowest quality of ChatGPT, purely copy paste from ChatGPTT into the exam system, led to these results. Clever students who would cheat a little bit, and I'm sure we'll speak about that, how we integrate GenAI into the teaching and learning experience, students will incorporate the production of GenAI into something. And so it's never going to be 100% GenAI.
Robert: Yes, that's the kind of interesting bit on this. So you were using just the raw response. And one of the questions that sort of struck to me as I was looking through your paper on this, did you, because how would you stop the fact that ChatGPT would produce exactly the same? So they could have ended up with 33 exact same, or did?
Peter: They were very similar. So if we submitted multiple submissions with the same question, we just used the regenerate button. We went in a position to kind of mimic how a student would potentially edit, so we just didn't. In some senses, students work is quite self-similar because they're taught the same material, they go to the same lectures, they read the same papers and things like that. But yeah, if you looked at the AI output, it was very self-similar to one another.
Robert: And you used simple prompts, so you're not, you know, experts in prompt engineering, so you weren't in some way operating at a higher level than a typical student way. It was, here is the exam question and give me an answer in a certain number of words. And it was as simple as that, was it?
Etienne: Yeah, that's right.
Robert: Wow. And did ChatGPT struggle with some of the word counts? Was the, did it make stuff up or, I suppose you didn't really worry about that. It was just copy and paste.
Peter: Struggled with word counts.
Etienne: Oh yeah, word count was wrong.
Peter: So basically in our prompts, we didn't actually state the real word count. So I think for short answer questions, we had to go under because it was a bit more verbose and then we struggled getting it to generate enough for a full essay. So we kind of adjusted the words we asked for. But it doesn't really plan its answers, so it doesn't really kind of set out with a word count in mind. It just kind of like trundles along and then when it's happy, it's finished, it's finished. But with that, we ended up getting questions which were, you know, roughly within the distribution of work counts of typical student answers.
Etienne: And if it stopped, we just say, please carry on. And that's it. Add another thousand words. Yeah, and we wouldn't edit that in any way. We wouldn't edit in any way. No, just add some more.
Robert: So standard exam question, you're using whatever the raw output of ChatGPT, you're putting it straight into… the exam software in there. And now after that, it goes to, I say professional, but trained markers. So it wasn't as if it was, you know, you were some way part of your experiment creating your own markers on this. This went into the normal exam marking system.
Peter: We had no control over who individually got that got the particular answers.
Robert: And those people are trained on how to mark, to look for plagiarism, similar answers, perhaps the introduction of AI, they're given a proper training on how to mark.
Etienne: The markers haven't been given actual training into detecting AI or things like that. But at the time, it was last AI was a lot of in the news or two years ago. AI was a lot in the news and so we had talked about it. And so there has been discussions of AI in various forums. The university did put some communication about we need to take care of that and, you know, be careful and things like that. But there was there weren't a formal training on that.
Peter: Indeed some of the markers on the scripts on AI, occasionally they were ours, but occasionally they were just not one of us.
Robert: Really? So in some of that, okay, we'll come back to that in a second. So trained markers going through it, human markers on all this, and there was some plagiarism software in play, because most universities have, I think only for the long form answers is that right, that they have a piece of software that will cross-check and the University of Reading uses that software and did in this case too.
Etienne: Yeah, that's right. So it went through the same pre-processing of the scripts. The software would give us a percentage of how much plagiarism there is in comparison to big databases of scripts that they have. But the university was not happy with the automated detection of AI and so we were not using the plugin for that particular software for that.
Peter: Yeah, at the time it was kind of like a beta piece of software, so the particular company were kind of testing it on universities in a way, saying, well, this is a beta piece of software, you can have a go with it if you want.
Robert: And just quickly on that, that wasn't very reliable, which is why it was beta and the university wasn't happy in turning it on, is that right? reduce too many false positives?
Peter: It's notoriously difficult to detect AI, both from a human perspective and also a software perspective. And the last thing in the world, you know, a university would want to do is falsely accuse students of cheating using AI when they're not. So by and large, these kind of systems, these AI detectors say, will kind of give you a percentage, you know, kind of, we think this is 23% AI written. What do you do with that? What do you do?
Robert: Do they give you any threshold if it's 90%? Do they say at that point, you know, we're really certain that AI is?
Peter: I mean, yes and no. I mean, obviously the system should be more certain then, but AI can be certain about things which are factually not correct. So it's very hard to do anything at all with that kind of number.
Robert: That's, thank you for covering that. And so we know that's the background to it. So I think everybody understands that it was done in a way that felt like a proper Turing test in this. Nobody was aware and it went through normal real life process. So let's just look at the results because I think that's what everybody is saying. Okay, I'm happy now because I think the results are eye popping in many ways and groundbreaking. And so let's just start with
First of all, that detection piece. How many of your 33 AI students and their various different modules were actually detected and how many actually sailed through the system undetected?
Peter: 94% were undetected.
Robert: 94% undetected.
Peter: And we adopted a kind of lax, well not lax, By detected, it just had to be that the submission was flagged as being suspicious in some way.
Robert: Oh, so really loose…
Peter: It didn't need to mention we think it's AI. It could be, hmm, this is just oddly written, and this is covering material not in the course. I think a student might have plagiarised or something like that. If we adopted a more stringent criteria and the flag had to mention AI again in any way, 96%.
Robert: Wow. And you probably have, I think most people would have thought somewhere between 5 and 10% of cheating going on anyway in an un-invigilated process. So it didn't seem that kind of number that you're talking about there being anything other than what you'd expect in a normal process anyway. So you've inserted a whole load of these AI scripts in there and it hasn't really probably changed the dial in any way. Did the detect the ones that were detected, it was just a bit, it just looked a bit odd, or say it all, or reasoned?
Peter: Yeah, it was just, they looked odd. I think we had one which said potentially we think this might be generated by AI.
Etienne: Yeah, I think when it was detected it was not necessarily that that particular piece looked odd, more that it looked odd in a context of the others as well. I remember this one marker who came back afterwards who told us, yeah, there was this one piece that I knew I could, I remember the structure that I had seen from somewhere else. Right. But because of workload and they didn't have the time to dig through to figure out what was before. And so, yeah, I think this was a.
Peter: Also, even if you start digging, it's very hard to prove.
Robert: To then prove it. So you've got a 94 to 96% chance of being undetected if you use AI on this. That's shocking, isn't it? I mean, doesn't that provide quite a big worry for the integrity of, and we'll come into how that influences the results. But the fact that you just can't really detect it in the very simple way that you were copying and pasting too. Were you surprised by that? Did you think it was going to be higher or you just weren't sure what was going to come out?
Peter: We knew the answers were going to be good because of the previous anecdotal reports and then we'd obviously in preparing for the project we've been having a go. I'm not sure we expected it to be as undetectable. We thought it was gonna be difficult, but I think I was a little surprised in just how difficult it was. Especially with the very naive approach that we took.
Robert: Yes. And so that's, I think that's an important part of this because what it probably means is that there's a load of use of AI by students at the moment and I think you alluded to that in your research as well, because if it's undetectable, as you've been able to prove then it probably is going on quite a lot. And that then leads then to the next bit was, okay, well, what were the results then? Because potentially if AI, two questions on this, one is, did it give a better result? But secondly, did it give such a better result that it skewed the overall distribution and therefore it was obvious that AI was being used? So what were the general results your AI usage?
Etienne: Overall, the AI design scripts fared much better than our regular students. On average by one... Half a class of K. Yeah, half class classification. So that's about a two one, that kind of setup. I think that students who would have used it would have benefited, and so would have had a better degree. Overall, when you look at the whole distribution of the whole grades for the whole modules, the distributions weren't very different from previous years, so it's a bit difficult to... So I don't think that we can say that students have used a lot of AI in that period.
Robert: Yes. And I think that was, for me, part of the fascinating bit of what your study revealed was that it was a sort of normal distribution of results there, but half a grade better.So if AI has been used and increasingly is being used, how that then plays out into the overall marking scheme is, oh, more people are getting two ones now. Pat on the back for the professors and the departments on this, because we must be doing something good now because our students are getting better results. So it didn't skew it in any way, it just provided half a grade more and so that would imply actually if you can be undetected and you're going to get half a grade better then why wouldn't you as a student be using this and that's you know that then really hits the integrity of the whole exam system around this and is that what you felt at the end of it when you saw these results oh my goodness we've got a big problem here.
Etienne: I mean you have to realize, been designed to get grades and exams are there to, as you know, demonstrate that a student has mastered content to then move on to different content and so it's really not in the interest of the students to use AI or cheat in any way. But the situation now is such that I think that students are under so much pressure and so on that they. I don't see why they wouldn't be using AI. And I'm sure we'll come to that, but it's actually, we probably would want them to use AI anyway at some point, right? Because that's what the employers would want. And so we have to integrate that in this particular way.
Robert: Yes, I think that's fascinating Etienne. And it's a really interesting point that you make on this, which is rather than push back and say, we need to find better ways to detect this and...We need to call out people who are cheating on this. We need to start thinking about, well, actually, what is it that we want to measure from a student's capability now? And how do we want to measure it rather than trying to sort of push the clock back and say, oh, AI is a real problem, so let's try and hold it back. Because fundamentally, that is not gonna help anybody.
And so we have to think about, well, what is it that we want to learn about a student's capability from the way that we then test them, rather than in the past, it used to be a recall of knowledge. But actually, AI can do that recall of knowledge better.
Peter: Certainly, our university, there's a lot of work going on revisiting unsupervised assessments because of this. AI is just a new tool. The genie's out of the bottle. It isn't gonna go away. I think we're, you know, we know we're not going to be able to detect it very well. You know, we were discussing right the way through the study that, you know, students are gonna be using AI in the workplace, you know. So just kind of putting your fingers in the air is just closing your eyes is- And hoping that it's gonna go away. It's gonna go away, please let it go away. You know, so it's, you know, unfortunately, it's gonna be a big piece of work for the educational sector and beyond. It's not just education.
Robert: Kind of conclusions from this were, as things stand, the integrity of an exam system that isn't invigilated is fundamentally at risk from AI as things stand today.
Peter: If AI isn't built into those assessments, if we're just using the same old unsupervised assessments, then yeah, a study in other work shows that yeah, that's going to be a problem. That's why we need to revisit those assessments.
Robert: We need to revisit it. And now that you have sort of brought this to light, and I think the interesting thing will come back to that as well as to how we might address it, the immediate, I'm sure, response from most universities is right, we've got to invigilate then. Is that how, what kind of response, since you've released your research, have you had people reaching out to you saying, oh no, Peter, what should we do? Or if they'd been trying to sort of pretend that your research wasn't quite as eye-popping as it was?
Etienne: It was eye-popping for many people. One way or another, some were pleased and it was sort of the emperor has no clothes situation. Others were a bit more reluctant, I would say. I think the obvious, let's go back to pen and paper, so is not going to be viable in many ways because there is an ever-growing number of students that need to take exams, there are constraints, the higher education sector is under massive, massive financial stress.
So, and we've gone so far ahead with home take home exams and so on to save costs, to increase diversity and inclusion and help the student experience generally. Going back to pen and paper, that is not gonna happen. What is likely to happen, however, and we will take more time is for the teaching body to understand how AI can be incorporated in the material as a tool for the students to use, and then to take for granted that students and actually ask the students, please use AI to generate this piece of work and then critique it, for example. And something like that. But that is going to take a long time because not everyone knows AI or an AI changes by the minute. So, and you have to realize that the timeline of exams at the university is really really long. The exams are set over a year and a half before the time when they're supposed to be. Right, so um, so there's we're constantly playing catch-up. Um so yeah, it will take some time, but um, I think that's...
Robert: So in the meantime, we need to give advice then to students as to what is good use and bad use of AI in exams. Otherwise, we're left in a mess of not being able to know who's used it, who's not used it, whether they've used it to get an extra advantage. And some people thought that it would be cheating and they might be caught and therefore didn't use it. So there seems to me an urgent need to give advice. And are you starting to think or have people ask you about
How do we set out what is good use of AI and what is bad use in an exam situation?
Peter:Yeah, I think Reading are really ahead of the curve there. So I'm involved in a lot of kind of policy work behind the scenes working out how assessments at the university are going to look, along with a lot of other people. And yeah, it's going to be a difficult process, but the feedback we've got from the paper from academics right up to kind of vice chancellor level of universities has been this is exactly what we needed. We needed this hard data to know that this, the extent of the problem and what we need to start thinking about going forward.
Robert: And just on that, is that a bit of a relief for you? Because I imagine doing a study that you know is going to potentially undermine the integrity of the university's exam system. It's the one output that people are expecting to get from a degree and they pay considerable money for that. And you've gone and secretly done a study here and you've kind of put your necks on the line a bit on this and you probably weren't sure how because I think testing the vulnerability is so important. But most people, unless it's an IT system, don't really want to do that because they're worried about what they might find. And so were you a bit worried about that, about how people might respond to what you find?
Etienne: He was, yes.
Peter: I was worried. I got less worried as things went on because we're scientists and we want hard evidence and we want data. And just because the answer might be unpalatable and cause us a lot of additional work, that isn't a reason just to, as we said before, just kind of close your eyes, put your fingers in your ears. But yeah, no, it was on our minds, certainly my mind for sure, that we, the paper could, whatever the results were, could potentially have a big impact. But we know it would be a good impact.
Etienne: Yes, we were supported by the university. Oh yeah. To do it, right. So in order to have approval to run the study, we had to...
Robert: Oh, you had to probably go through an ethics check and all.
Etienne: The full story is we tried to get ethics, but because this is more a quality assurance process, we had to do more than that. We had to go through the provisor for teaching and learning, sign off on this, and then everyone down the line. Yes, the number of people who have to sign off on this is.
Peter: It was great though. I think other universities wouldn't have been as brave. I think. Yes. I think Reading was, you know, it was great to be allowed to do it. And I think the evidence and the feedback we've got back has just been overwhelmingly positive.
Robert: And from other universities too.
Peter: Oh yeah. They wanted to know what you did. We just got unsolicited emails from folks saying, this is exactly what the sector needed. You know, thanks for doing this.
Etienne: From higher management, providers, from other universities, emailing us saying, thank you very much, we've just discussed your paper in a board meeting and this is a game changer.
Robert: Yes. Oh, I mean, I think it's fantastic. And it's funny, you don't know when you're preparing these things and you think as a study that it comes at a point in time, which is quite pivotal in both education but also for what happens after education as people come into graduate roles. As you alluded to, the implications of what you discovered is not just about the university sector but also how do we assess people once they leave university and apply for jobs too, and that they're going to be using AI in jobs as well.
Peter: I mean, we always thought, we always focused on the bigger picture in a way. Like, you know, we called it a case study because it kind of was a case study. It happened to be in an educational setting because we happen to be in an educational setting. But, you know, we were always talking about how, you know, the issue of AI is going to really kind of fundamentally change how we consume information on the, you know, for example, on the internet, you read a piece of news, you know, we're living in, I've said this a few times now, I'm getting bored, you know, living in this kind of, you know, what's Trump's phrase, fake news and alternative truths, you know, I think we're just, you know, going to be, have to be more discerning consumers of information in general, so we always were just looking at that bigger picture.
Robert: Yes, and I think it's great to kind of conclude on that piece that we've got to embrace AI. If we embrace AI in the right way, we can then think about how that will support the way that society consumes information now, which is very different in an AI world from what it was before. One of the biggest things being what's true and what's not true and how do you discern that and that's really interesting because university education used to be you're going to learn about the truth of the world to some extent, that's what you pick up from it because you won't be able to pick it up in any other way other than through reading subsequently.
Whereas now that information is at your fingertips so it changes the, oh acquire knowledge to then be able to, in some way, use it or move the world forward to, I need to discern now what is real information and what is fake news and how do I discern that? It's a different type of problem-solving that we'll want education to support. And so just on that note, just how are the things that you think that a psychology degree might change.
Etienne: It's a very broad question. Just on the way here, we were discussing creating a new program that would incorporate AI.
Peter: Yes. I think we've always had a mix of different types of assessments, even before AI, coursework, presentations, podcasts, things like that. And I think we'll continue having that mix of different assessments. I think there'll be a really important place for supervised assessments of some description, whatever they may be, not necessarily pen and paper exams, where we can guarantee for certain that students haven't had access to AI. Then there will be those assessments where, as Etienne has said, we would have to assume students would be using AI regardless, so we need to build it in. Those assessments will just look different.
The big question, which I would be very rich, if I knew the answer to was, exactly how will we design those? What exactly will they look like? And that's just a tough question. We have to figure that out. We just had to work through it. AI, I think, took computer scientists by surprise, yeah, as to how good it had got. So it wasn't as if the educational sector were the only ones who were kind of behind the curve. I think it was really surprised everyone.
Robert: I think it has, and thank you for sharing everything that you have today. I think it's an amazing piece of research. I'm sure we will look back in time and people will refer to what you revealed on this as being a turning point when people started to realize how significant AI is going to mean for us going forward and there is much more to it than some of the hype that people assumed that it would not live up to. But you've shown that there are some really big things that we need to understand and think about and that we may not have all the answers now but we need to be figuring out and talking and discussing and thinking about the changes we need to make because if we don't make them we're going to end up in a real mess. And so we have to start planning and thinking about that now. But really delighted to have you on the podcast and thank you for coming on.
Peter: Thanks for having us.
Read Next
Sign up for our newsletter to be notified as soon as our next research piece drops.
Join over 2,000 disruptive TA leaders and get insights into the latest trends turning TA on its head in your inbox, every week
Sign up for our newsletter to be notified as soon as our next research piece drops.
Join over 2,000 disruptive TA leaders and get insights into the latest trends turning TA on its head in your inbox, every week