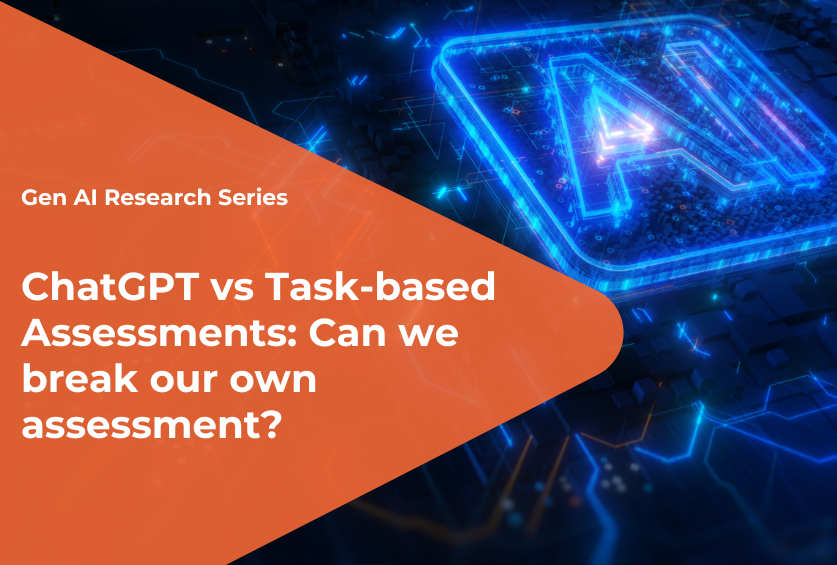
So far, our research series has uncovered how candidates can use ChatGPT to complete three types of traditional, question-based selection tools: Aptitude Tests, Situational Judgement Tests, and Personality Assessments. We’ve shown how ChatGPT:
- Outperforms 98.8% of human candidates in verbal reasoning tests
- Scores in the 70th percentile on Situational Judgement Tests
- Can ace question-based Personality Assessments for any role, by simply reading the job description.
However, we were left with one last question: if ChatGPT can achieve high scores in traditional, question-based psychometric assessments; how does it perform on an interactive and visual Task-based Assessment?
In this fourth and final research piece, our team set out to answer the following questions:
- Can a candidate use ChatGPT to outperform the average score on a Task-based Assessment?
- Is it possible to translate an interactive and visual assessment task into text, so that ChatGPT can understand it and give meaningful recommendations?
- Could other forms of Generative AI complete a Task-based Assessment?
Yes, you read that right. In this piece of research, with the help of two independent (and very tenacious) UCL postgraduate researchers, we were trying to see if we could break our own Task-based Assessment.
Throughout this post, we’ll lay out our methodology and present our findings. But for those seeking a quick peek, here are the headlines...
TL;DR
- ChatGPT could not complete a Task-based Assessment using text inputs — because of the interactive, visual nature of tasks, the only way of explaining the assessment to ChatGPT via text is to manually type out what’s happening in the task and see if it could respond. That took too long to be practical and did not improve candidate scores in any way.
- Image-to-text software does not help to explain the tasks to ChatGPT — this tech works by scanning a screen and creating text, which can then be pasted into ChatGPT. However, the only text available to scan in Task-based Assessments are the instructions, and all ChatGPT could do with those was rewrite them.
- Image recognition software does not help candidates in completing tasks — at the time of writing, ChatGPT does not read images; so we tried uploading images of the tasks to Google Bard instead. Bard struggled to understand the tasks or make any useful recommendations. The dynamic and interactive nature of the tasks made them too complex for image recognition software.
- We tried asking ChatGPT to write the code for a bot to complete a task, but this did not work either –– the results were very poor and even using more complex Generative AI tools to do this did not yield better results.
- These findings lead us to conclude that Task-based Assessments are one of the most robust selection methods on the market today for being able to see a candidate’s true potential to succeed in a role.
Now into the details...
What is a Task-based Assessment and how does it work?
A ‘Task-based’ Assessment is defined as follows: there is no language element to the activity the candidate is being asked to perform, or to their response.
In the early days, the description given to this type of assessment was ‘game-based’ because some of the features included mirrored those in gaming. For example, points, guided stories and badges.
Since those early designs, nearly 10 years ago, these types of assessment have evolved, losing any ‘game-like’ elements such as a story or points. Though they do retain their interactive and engaging nature.
This enables TA teams and hiring managers to capture thousands of data points on candidates’ potential to succeed in a role based on their actions — not their words.
The Task-based Assessment format can be used to assess both Personality and Aptitude. For full transparency, Arctic Shores classifies itself as a Task-based psychometric assessment provider. We assess Personality and Workplace Intelligence, which is our more expansive interpretation of Aptitude; covering things like Learning Agility and facets of Emotional Intelligence.
How do employers benefit from using tasks?
Capturing how candidates naturally respond to carefully designed tasks allows the assessment vendor to gather primary evidence of a candidate’s behaviour. For example, you can measure a candidate’s actions to predict how well they take ownership and meet deadlines — rather than relying on secondary evidence, i.e. the candidate telling or reporting how they might behave.
Task-based Assessments deliver this primary evidence right to the start of the selection process. This allows employers, at the earliest stages of the selection process, to more accurately assess things which are harder to confirm through questions; like risk appetite, learning ability, resilience, and how candidates perceive emotions.
How do Task-based Assessments compare with traditional question-based assessments?
Below, to the left is a traditional, question-based assessment. On the right is a Task-based Assessment. Both of them aim to reveal a candidate’s personality profile, but they do so in very different ways.
Question-based assessments (whether purely personality-focused or Situational Judgement Tests) rely on the candidate answering authentically about what they’re like or how they would act. They complete a series of multiple-choice questions — ranking how strongly they agree or disagree with the statements.
The challenge with this approach is that a candidate might not have the self-awareness to accurately report what they are like; or may present a distorted view as they try to second-guess the ‘right’ answer or respond to what they might aspire to be (otherwise known as Social Desirability Bias, which was discussed in more detail in the previous blog: ChatGPT vs Personality Assessments).
Task-based Assessments, on the other hand, rely on interactive visual tasks. As the candidate completes the task, they’re measured on their actual behaviours. They tend to provide a better experience for the candidate than many of the less engaging question-based assessments that are available. (90% of candidates report that they enjoy the Arctic Shores assessment.)
For example, let’s say that you want to measure a candidate’s performance under pressure. A question-based assessment, especially a Situational Judgement Test, would simply ask them how well they think they perform under pressure. But this is open to all kinds of distortions. From Social Desirability Bias, which affects all candidates, to Stereotype Threat, which disproportionately affects candidates from diverse backgrounds (Hausdorf, LeBlanc, Chawla - 2003).
A Task-based Assessment, on the other hand, will test a candidate’s performance by asking them to complete a task with varying degrees of pressure and comparing that to a general population group. This gives a more accurate insight into that personality trait. Plus, it is based on thousands of data points — versus a few hundred for question-based assessments.
The same is true for a Task-based assessment of cognitive ability, compared to a traditional question-based aptitude test.
While traditional Aptitude Tests tend to use multiple choice — Task-based aptitude tests allow candidates to build their answers step by step. It’s a much more engaging process than a multiple-choice questionnaire.
Arctic Shores’ latest Task-based aptitude test also overhauls the traditional scoring method, which relies on a binary “right” or “wrong” answer. This approach misses out on the benefits of capturing any ‘workings out’ from the candidate — in particular, screening in candidates who get most of the answer correct.
In fact, our initial research revealed that 20% of candidates who ran out of time in a traditional aptitude test actually worked out 90% of the answer.
The education system moved away from binary scoring many years ago and now lets students build their solution; with each step counting towards their grade. Taking this approach in aptitude tests allows forward-thinking employers to accurately screen in candidates who get most of their answers correct. Just like you would in an interview.
To summarise, you can think of the difference between question-based and Task-based like this: if you wanted to find out if someone can drive, would you rather ask them or get them to drive? A Task-based approach puts candidates in the driving seat. We collect data on their actual behaviours — like how they respond to hazards or what happens when they have to change direction. This prevents the assessment from being coached, guessed or faked. And it gives TA teams the best possible insights into candidates’ ability to succeed in the role.
Known challenges with Task-based Assessments
As a newer and more innovative selection tool, Task-based Assessments overcome many of the challenges faced by traditional question-based tests. For example, adverse impact on protected groups arising from Stereotype Threat (Hausdorf, LeBlanc, Chawla - 2003). As a result, the known challenges with Task-based Assessments are less about the assessment design — and more to do with implementation.
Rolling out an innovative approach like this requires planning and support from experienced vendors. In particular, securing buy-in from hiring managers on a new scientific approach needs nuanced messaging. While this issue is certainly not unique to Task-based Assessments, it can be more pronounced.
After all, it’s not immediately clear how pressing buttons or clicking screens reveals deep insights into candidates’ personalities and levels of workplace intelligence. Whereas the text-based question and answer format used in traditional assessments is much easier to comprehend.
This is why TA Teams should always check that any Task-based Assessment provider is capable of supporting the implementation of the assessment to get the maximum return on their investment.
Our research methodology
For this research project, we tested every single task in our assessment against ChatGPT — and, as we’ll go on to show, other Generative AI tools too.
Traditional question-based psychometric assessments all have a large text component to them. They rely on words to ask questions, and to collect answers. So when it came to our research, our first consideration was how to get a good enough description of a task into ChatGPT so it could process the information.
In previous research, we used the ChatGPT iPhone app to convert images to text, before creating a bank of questions for standardised testing. We then focused on coming up with the most effective prompting styles, and testing them against all available GPT models — i.e. GPT3.5 (free) and GPT-4 (paid). To see more detail on this approach, please refer back to our other blogs on ChatGPT vs Aptitude Tests, SJTs and Personality Assessments.
When we tried this same approach with our Task-based Assessment, however, we instantly hit a roadblock. Our assessment is not text or question-based. It’s visual. And that meant we had to get creative — and test these three hypotheses to try and break our own assessment.
Using image-to-text to scan the instructions
Our tasks contain no text. But the instructions that precede the tasks do:
This meant that candidates could use the same approach that we’ve outlined in previous blogs to quickly and easily scan in the instructions to ChatGPT. We were sceptical this would work because the instructions are general but the tasks are unique. This means in theory there is no standardised approach that can be inferred for all tasks. But we wanted to test this hypothesis to make sure.
Looking to other generative AI models
At the time of writing, ChatGPT can’t process images; but Google’s generative AI model (Bard) can. The idea of this piece of research was to test whether a candidate could take a picture of the task, show it to Bard, and get instructions on how to complete it.
Creating a bot to complete the assessment
Okay, this one sounds a bit Sci-Fi — and we admit, it’s not likely that a candidate will be able to do this. But, in the interests of making sure our assessment would stay robust vs ChatGPT, we wanted to test all of the possibilities. This meant identifying whether someone with the right skills could create an automated bot to complete our assessment. For this one, we recruited an external software engineer to create the bot and then unleash it on our assessment.
The results — can generative AI be used to complete a Task-based Assessment? Three findings…
1. ChatGPT could not complete the assessment by translating the instructions using an image-to-text scanner
As we suspected, this approach was not effective. The process of scanning the instructions, converting them to text, and then pasting them into ChatGPT was relatively straightforward, as we found in earlier tests.
The blocker came from how ChatGPT used the instructions. Rather than making useful inferences, it simply rephrases the wording of the instructions. This provided no helpful additional information for candidates on how to complete the task, over and above what’s already provided.
In one sense, it’s exactly the same as if the candidate had a very knowledgeable friend helping them to make sense of the instructions; which is no bad thing given the instructions are supposed to be as helpful as possible. It’s the tasks that assess the candidate.
Below is an example of where ChatGPT rephrased the instructions:
As you can see, the response simply elaborates on the original instructions, rather than deducing any additional information.
The takeaway here is that ChatGPT can give advice, but it can’t actually complete the assessment for a candidate. It can’t interact with the screen, or press any buttons. And where there are no right or wrong answers, following anyone else’s advice won’t do candidates any favours. That’s because ChatGPT (or any other advisor) would have to both understand what qualities the employer is looking for — and exactly how to interact with the task at a micro level to generate scores in line with the employer’s requirements. Remember that each task collects thousands of data points on the candidate’s interactions.
2. Generative AI could not complete the assessment using image recognition software
To test this hypothesis, we went outside of ChatGPT and used an alternative generative AI model called Bard. As we explained above, Google’s Bard allows you to paste in images, which then reads like text. On the face of it, this posed a bigger problem for Task-based Assessments than the first test. The robustness of Task-based Assessments comes from being unreadable by ChatGPT — so what happens when a generative AI tool can suddenly read images?
What we’ve discovered is that this sort of image recognition technology is very good at picking out a single large image. (Imagine asking your phone to find all of your pictures with a dog in them.) But it’s no good at deciphering multiple shapes and elements on a screen…it’s just too complex for the AI model — it’s not what it’s been designed to do.
In other words, it struggles to parse out individual elements — and that’s exactly what a Task-based Assessment asks candidates to do: it gives them lots of elements and asks them to process them.
Take the example below. (N.B. Some parts are blurred out to protect the integrity of the task):
As you can see, the answer looks like it could work. Bard identified the need for certain shapes, for example. But on closer inspection, it has chosen completely the wrong shapes. There is no star included in the list of available options.
What this speaks to is a misalignment between what the image recognition technology is created to do and how the tasks are constructed.
Image recognition is designed to read static images. But tasks are not static. They are dynamic, moving puzzles that change frequently. So any image recognition would have to read multiple consecutive snapshot images to get a picture of the task as a whole, to then be able to respond and influence the results positively. The sheer volume of times this would need to be repeated to complete the task makes this approach unviable. As the research reveals, the best Bard can do is ‘hallucinate’ an answer — i.e. get it completely wrong.
In the above example, Bard ‘hallucinates’ a star when in fact that’s not one of the available shapes to choose from.
3. Generative AI could not complete the assessment by creating a bot
For this part of the research, we recruited an external software developer to create a bot from code that was generated by ChatGPT. This allowed us to test the next level of sophistication. Further details of how the bot was created can be provided on request.
The bottom line is that the bot could not complete a Task-based Assessment, for the same reason that the other two approaches failed: it wasn’t able to process that level of complex visual data.
For this test, the bot used a technique called Optical Character Recognition, or OCR. It became apparent very quickly that just working from a single image was a major blocker to the bot completing the tasks. Again, the complexity of the task overwhelmed the bot in the same way as Bard had been overwhelmed — making it impossible to complete the assessment, let alone outperform human candidates.
Another point to note here is that, as we’ve explained in previous blogs in this series, it can be very hard to identify when ChatGPT is being used by candidates to complete traditional selection methods. That’s because ChatGPT’s outputs are probabilistic — i.e. they have a degree of randomness baked into them. This randomness makes its outputs sound very human.
On the other hand, wherever bots are involved, detection becomes much easier. As a result, we concluded that this option is not viable for completing a Task-based Assessment at this time.
Candidates can’t use generative AI to complete Task-based Assessments — what does this mean for TA Teams?
This research leads us to conclude that candidates cannot use ChatGPT or other generative AI tools to complete an interactive and visual Task-based Assessment. What’s more, even the introduction of specialist coding ability doesn’t overcome the basic challenge: there is currently no way to explain a complex visual assessment to a Generative AI Model like ChatGPT or Bard.
Of course, no one can predict what the future might bring — and we’re committed to monitoring this changing landscape as the technology evolves. If we discover any new tools being used extensively, we’ll be the first to test them and publish what we find.
TA leaders now have three ways of responding to candidates using Generative AI.
Detect
Continue using traditional, question-based psychometric assessments, but try to use additional tools to detect if candidates are using Generative AI to complete them. Given that the detection-proof method of turning up the Temperature on ChatGPT often results in worse performance, this could still seem like a viable option.
But the main challenge with this approach is that no ChatGPT detection models have been shown to work effectively as of today, with some even reporting that 2 in 10 times these detection methods produce a false positive, meaning you risk falsely accusing candidates of cheating, potentially harming your employer brand.
It’s also worth noting that given how quickly the underlying language models change and improve, there’s a chance these detection methods could quickly become out of date.
Deter
Attempt to prevent candidates by using (already unpopular) methods like video proctoring, which have been around for several years but received strong pushback from candidates. These methods are also likely to increase candidate anxiety, shrinking your talent pool further (Hausdorf, LeBlanc, Chawla 2003).
Simpler methods like preventing multiple tabs from being open at a time or preventing copy and paste from within a browser are options, but even these can be easily bypassed with a phone.
For example, candidates can use the ChatGPT iPhone app to scan an image, get a response, and input the suggested answer into a computer in just a few seconds.
Design
Look for a different way to assess how a candidate would really behave at work and in a way that cannot be completed by ChatGPT. The starting point is to choose an assessment type that isn’t based on language, and so can’t easily be completed by large language AI models like ChatGPT. Based on our research, interactive, Task-based Assessments currently prove much more robust and less susceptible to completion by ChatGPT because they create scores based on actions, not language. They also give you the added benefit of being able to assess Personality and Workplace Intelligence in one assessment.
If you’re looking to measure communication skills, you might also consider a more practical way to assess that using a video screening platform.
Where to go from here
Given the pace at which these AI models are moving, what is true today may not remain true for very long. So while you think about adapting the design of your Selection process, it’s also important to stay up-to-date with the latest research.
We’re continuing to explore the impact of ChatGPT across the wider assessment industry. You can view the other blogs in this research series here, or sign up for our newsletter to be notified when our next piece of research drops.
The focus of our next research piece? We’ve surveyed 2,000 students and recent graduates to understand how students’ use of ChatGPT will make traditional selection processes redundant in Early Careers. (We’re also launching a podcast series exploring this topic, which you’ll be able to listen to very soon.)
Subscribe below and be the first to know which assessment format you should choose to future-proof your selection process.
Sign up for our newsletter to be notified as soon as our next research piece drops.
Join over 2,000 disruptive TA leaders and get insights into the latest trends turning TA on its head in your inbox, every week
Read Next
Sign up for our newsletter to be notified as soon as our next research piece drops.
Join over 2,000 disruptive TA leaders and get insights into the latest trends turning TA on its head in your inbox, every week
Sign up for our newsletter to be notified as soon as our next research piece drops.
Join over 2,000 disruptive TA leaders and get insights into the latest trends turning TA on its head in your inbox, every week